AI-Driven Innovation in 5G: Real-Time Neural Receivers Pave the Way
Felix Pinkston Sep 04, 2024 09:04
NVIDIA introduces neural network-based wireless receivers, enhancing 5G NR with real-time AI capabilities. Discover the future of AI-RAN and 6G research.
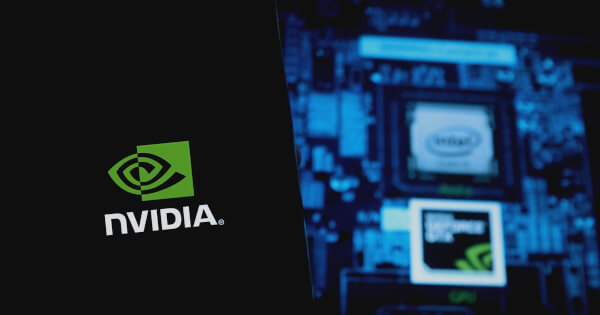
Advancements in 5G New Radio (5G NR) wireless communication systems are being driven by cutting-edge AI technologies, according to a detailed report from the NVIDIA Technical Blog. These systems rely on highly optimized signal processing algorithms to reconstruct transmitted messages from noisy channel observations in mere microseconds.
Historical Context and Rediscovery of Algorithms
Over the decades, telecommunications engineers have continuously improved signal processing algorithms to meet the demanding real-time constraints of wireless communications. Notably, low-density parity-check (LDPC) codes, initially discovered by Gallager in the 1960s and later rediscovered by David MacKay in the 1990s, now serve as the backbone of 5G NR.
The Role of AI in Wireless Communications
AI's potential to enhance wireless communications has garnered significant attention from both academia and industry. AI-driven solutions promise superior reliability and accuracy compared to traditional physical layer algorithms. This has paved the way for the concept of an AI radio access network (AI-RAN).
NVIDIA's Research Breakthroughs
NVIDIA has developed a prototype neural network-based wireless receiver that replaces parts of the physical layer signal processing with learned components. Emphasizing real-time inference, NVIDIA has released a comprehensive research code available on GitHub, enabling researchers to design, train, and evaluate these neural network-based receivers.
Real-time inference is facilitated through NVIDIA TensorRT on GPU-accelerated hardware platforms, providing a seamless transition from conceptual prototyping to commercial-grade deployment.
From Traditional Signal Processing to Neural Receivers
Neural receivers (NRX) combine channel estimation, equalization, and demapping into a single neural network, trained to estimate transmitted bits from channel observations. This approach offers a drop-in replacement for existing signal processing algorithms, achieving inference latency of less than 1 ms on NVIDIA A100 GPUs.
5G NR Standard Compliance and Reconfiguration
Integrating NRX into the 5G NR standard presents several challenges. The NRX architecture must adapt dynamically to support different modulation and coding schemes (MCS) without re-training. It also supports arbitrary numbers of sub-carriers and multi-user MIMO configurations.
Training is conducted in urban microcell scenarios using randomized macro-parameters to ensure resilience under various channel conditions. Site-specific fine-tuning further enhances performance post-deployment.
Performance Under Real-Time Constraints
Deploying AI algorithms in real-time systems requires meeting strict latency requirements. The NRX architecture is optimized using TensorRT on NVIDIA A100 GPUs to ensure realistic latency measurements and eliminate performance bottlenecks.
The NRX can be reconfigured to adapt to changing hardware platforms or system parameters, maintaining competitive performance even under real-time constraints.
Site-Specific Fine-Tuning
AI-RAN components can undergo site-specific fine-tuning, refining neural network weights after deployment. This process leverages AI-based algorithms and software-defined RANs to extract training data from active systems. Fine-tuning enables smaller NRX architectures to perform at the level of larger, universally pre-trained models, saving computational resources while maintaining superior error-rate performance.
Advancing Towards 6G Research
Neural receivers not only replace existing receiver algorithms but also enable novel features like pilotless communications and site-specific retraining. End-to-end learning approaches can remove pilot overhead, increasing data rates and reliability.
Although these innovations are not yet compliant with the 5G NR standard, they indicate how AI may drive novel 6G features for higher reliability and throughput. For additional details, visit the NVlabs/neural_rx repository on GitHub.
Image source: Shutterstock