Anthropic Explores Challenges and Methods in AI Red Teaming
Rebeca Moen Jun 13, 2024 09:10
Anthropic discusses insights and challenges in AI red teaming, aiming for standardized practices.
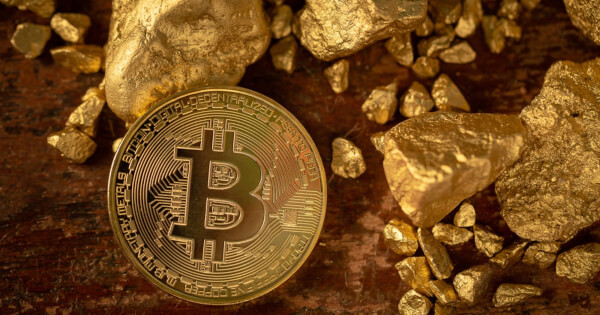
Anthropic has detailed insights from a sample of red teaming approaches used to test their AI systems, according to a recent post on their website. This practice has allowed the company to gather empirical data on appropriate tools for various situations and the associated benefits and challenges of each approach.
What is Red Teaming?
Red teaming is a critical tool for improving the safety and security of AI systems. It involves adversarially testing a technological system to identify potential vulnerabilities. Researchers and AI developers employ a wide range of red teaming techniques to test their AI systems, each with its own advantages and disadvantages.
The lack of standardized practices for AI red teaming further complicates the situation. Developers might use different techniques to assess the same type of threat model, making it challenging to objectively compare the relative safety of different AI systems.
Domain-Specific Expert Red Teaming
Domain-specific expert teaming involves collaborating with subject matter experts to identify and assess potential vulnerabilities or risks in AI systems within their area of expertise. This approach brings a deeper understanding of complex, context-specific issues.
Policy Vulnerability Testing for Trust & Safety Risks
High-risk threats, such as those that pose severe harm to people or negatively impact society, warrant sophisticated red team methods and collaboration with external subject matter experts. Anthropic adopts a form of red teaming called “Policy Vulnerability Testing” (PVT) within the Trust & Safety space. This involves in-depth, qualitative testing conducted in collaboration with experts on a variety of policy topics covered under their Usage Policy.
Frontier Threats Red Teaming for National Security Risks
Anthropic has continued to build out evaluation techniques to measure “frontier threats” that may pose a consequential risk to national security, such as Chemical, Biological, Radiological, and Nuclear (CBRN) threats, cybersecurity, and autonomous AI risks. This work involves testing both standard deployed and non-commercial versions of their AI systems to investigate risks in real-world settings.
Multilingual and Multicultural Red Teaming
Most red teaming work takes place in English and typically from the perspective of people based in the United States. To address this lack of representation, Anthropic has partnered with Singapore’s Infocomm Media Development Authority (IMDA) and AI Verify Foundation on a red teaming project across four languages (English, Tamil, Mandarin, and Malay) and topics relevant to a Singaporean audience.
Using Language Models to Red Team
Using language models to red team involves leveraging AI systems to automatically generate adversarial examples and test the robustness of other AI models. This approach can complement manual testing efforts and enable more efficient and comprehensive red teaming.
Automated Red Teaming
Anthropic employs a red team/blue team dynamic, where a model generates attacks to elicit target behavior (red team) and then fine-tunes a model on those outputs to make it more robust (blue team). This iterative process helps devise new attack vectors and ideally make systems more resilient to a range of adversarial attacks.
Red Teaming in New Modalities
Red teaming in new modalities involves testing AI systems that can process and respond to various forms of input, such as images or audio. This helps identify novel risks and failure modes associated with these expanded capabilities before systems are deployed.
Multimodal Red Teaming
The Claude family of models can take in visual information and provide text-based outputs, presenting potential new risks. Pre-deployment red teaming is critical for any release, especially those that include new model capabilities and modalities.
Open-Ended, General Red Teaming
Crowdsourced Red Teaming for General Harms
Anthropic has engaged crowdworkers in a controlled environment to use their own judgment for attack types. This approach allows for a broader cross-section of society to test AI systems for various risks.
Community Red Teaming for General Risks and System Limitations
Efforts such as DEF CON’s AI Village have engaged a broader cross-section of society in testing publicly deployed systems. Anthropic hopes that such challenges can inspire a more diverse group of people to get involved in AI safety efforts.
From Qualitative Red Teaming to Quantitative Evaluations
Red teaming practices serve as a precursor to building automated, quantitative evaluation methods. The goal is to turn red teaming results into something that creates compounding value for the organization. This involves an iterative loop of assessing an AI model for various risks, implementing mitigations, and testing the efficacy of those guardrails.
Policy Recommendations
To support further adoption and standardization of red teaming, Anthropic encourages policymakers to:
- Fund organizations such as the National Institute of Standards and Technology (NIST) to develop technical standards for red teaming AI systems.
- Fund independent government bodies and non-profit organizations that can partner with developers to red team systems for potential risks.
- Encourage the development of a market for professional AI red teaming services and establish a certification process for these organizations.
- Encourage AI companies to allow third-party red teaming of their AI systems by vetted outside groups under safe conditions.
- Encourage AI companies to tie their red teaming practices to clear policies on the conditions they must meet to continue scaling the development and release of new models.
Conclusion
Red teaming is a valuable technique for identifying and mitigating risks in AI systems. By investing in red teaming, organizations can work towards building AI systems that are safe and beneficial to society. It is one of several tools in a larger effort to ensure AI is developed thoughtfully and with robust safeguards in place.
For more details, visit the original post on Anthropic.
Image source: Shutterstock