NVIDIA Launches Nemotron-4 340B for Synthetic Data Generation in AI Training
Iris Coleman Jun 15, 2024 04:53
NVIDIA unveils Nemotron-4 340B, an open synthetic data generation pipeline optimized for large language models.
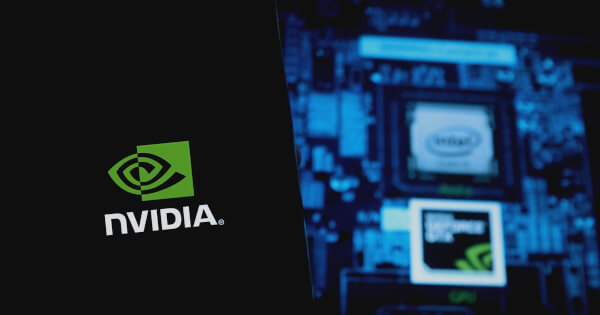
NVIDIA has introduced Nemotron-4 340B, a new family of models designed to generate synthetic data for training large language models (LLMs) across various industries, including healthcare, finance, manufacturing, and retail, according to the NVIDIA Blog.
Navigating Nemotron to Generate Synthetic Data
High-quality training data is crucial for the performance and accuracy of custom LLMs. However, obtaining robust datasets can be costly and challenging. Nemotron-4 340B aims to address this by providing developers with a free and scalable way to generate synthetic data through a permissive open model license.
The Nemotron-4 340B family includes base, instruct, and reward models optimized to work with NVIDIA NeMo and NVIDIA TensorRT-LLM. These models form a pipeline to generate synthetic data used for training and refining LLMs. Developers can download Nemotron-4 340B from Hugging Face and will soon be able to access the models at ai.nvidia.com.
Fine-Tuning With NeMo, Optimizing for Inference With TensorRT-LLM
Utilizing open-source frameworks such as NVIDIA NeMo and NVIDIA TensorRT-LLM, developers can optimize the efficiency of their instruct and reward models to generate synthetic data and score responses. All Nemotron-4 340B models are optimized with TensorRT-LLM to leverage tensor parallelism, enabling efficient inference at scale.
Nemotron-4 340B Base, trained on 9 trillion tokens, can be customized using the NeMo framework to fit specific use cases or domains. This fine-tuning process benefits from extensive pretraining data, yielding more accurate outputs for specific downstream tasks.
Customization methods available through the NeMo framework include supervised fine-tuning and parameter-efficient fine-tuning methods such as low-rank adaptation (LoRA). Developers can also align their models with NeMo Aligner and datasets annotated by Nemotron-4 340B Reward to ensure accurate and contextually appropriate outputs.
Evaluating Model Security and Getting Started
The Nemotron-4 340B Instruct model has undergone extensive safety evaluation, including adversarial tests, and performed well across various risk indicators. However, users should still carefully evaluate the model’s outputs to ensure the synthetically generated data is suitable, safe, and accurate for their specific use case.
For more detailed information on model security and safety evaluation, users can refer to the model card. Nemotron-4 340B models can be downloaded via Hugging Face. Researchers and developers interested in the underlying technology can also review the research papers on the model and dataset.
Image source: Shutterstock