NVIDIA Surpasses 1,000 TPS/User with Llama 4 Maverick and Blackwell GPUs
Lawrence Jengar May 23, 2025 02:10
NVIDIA achieves a world-record inference speed of over 1,000 TPS/user using Blackwell GPUs and Llama 4 Maverick, setting a new standard for AI model performance.
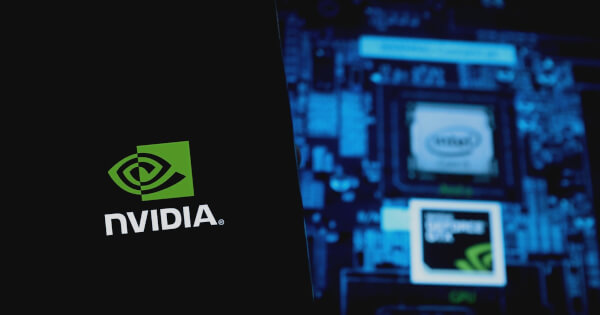
NVIDIA has set a new benchmark in artificial intelligence performance with its latest achievement, breaking the 1,000 tokens per second (TPS) per user barrier using the Llama 4 Maverick model and Blackwell GPUs. This accomplishment was independently verified by the AI benchmarking service Artificial Analysis, marking a significant milestone in large language model (LLM) inference speed.
Technological Advancements
The breakthrough was achieved on a single NVIDIA DGX B200 node equipped with eight NVIDIA Blackwell GPUs, which managed to handle over 1,000 TPS per user on the Llama 4 Maverick, a 400-billion-parameter model. This performance makes Blackwell the optimal hardware for deploying Llama 4, either for maximizing throughput or minimizing latency, reaching up to 72,000 TPS/server in high throughput configurations.
Optimization Techniques
NVIDIA implemented extensive software optimizations using TensorRT-LLM to fully utilize the Blackwell GPUs. The company also trained a speculative decoding draft model using EAGLE-3 techniques, resulting in a fourfold speed increase compared to previous baselines. These enhancements maintain response accuracy while boosting performance, leveraging FP8 data types for operations like GEMMs and Mixture of Experts, ensuring accuracy comparable to BF16 metrics.
Importance of Low Latency
In generative AI applications, balancing throughput and latency is crucial. For critical applications requiring rapid decision-making, NVIDIA's Blackwell GPUs excel by minimizing latency, as demonstrated by the TPS/user record. The hardware's ability to handle high throughput and low latency makes it ideal for various AI tasks.
Cuda Kernel and Speculative Decoding
NVIDIA optimized CUDA kernels for GEMMs, MoE, and Attention operations, utilizing spatial partitioning and efficient memory data loading to maximize performance. Speculative decoding was employed to accelerate LLM inference speed by using a smaller, faster draft model to predict speculative tokens, verified by the larger target LLM. This approach yields significant speed-ups, particularly when the draft model's predictions are accurate.
Programmatic Dependent Launch
To further enhance performance, NVIDIA utilized Programmatic Dependent Launch (PDL) to reduce GPU idle time between consecutive CUDA kernels. This technique allows overlapping kernel execution, improving GPU utilization and eliminating performance gaps.
NVIDIA's achievements underscore its leadership in AI infrastructure and data center technology, setting new standards for speed and efficiency in AI model deployment. The innovations in Blackwell architecture and software optimization continue to push the boundaries of what's possible in AI performance, ensuring responsive, real-time user experiences and robust AI applications.
For more detailed information, visit the NVIDIA official blog.
Image source: Shutterstock