NVIDIA Unveils NeMo Retriever Microservices to Enhance AI Accuracy and Throughput
Lawrence Jengar Jul 24, 2024 01:25
NVIDIA introduces NeMo Retriever NIM microservices, improving AI accuracy and throughput, integrated with platforms like Cohesity and NetApp.
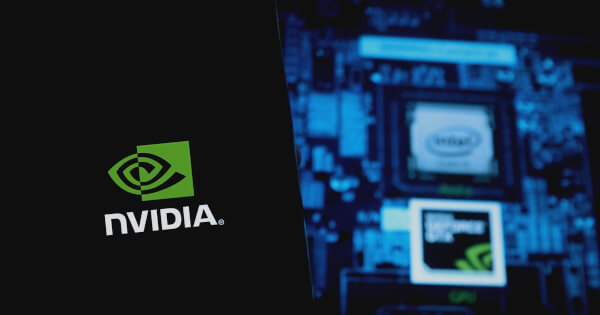
NVIDIA has announced the launch of its new NeMo Retriever NIM (NeMo Inference Microservices) designed to significantly enhance the accuracy and throughput of Large Language Models (LLMs) in AI applications. These microservices aim to help developers access and utilize proprietary data more efficiently, thereby generating more accurate and relevant responses for AI-driven tasks, according to the NVIDIA Blog.
Boosting AI Accuracy with NeMo Retriever
The NeMo Retriever NIM microservices are production-ready and designed for retrieval-augmented generation (RAG). This new suite of tools allows enterprises to scale AI workflows with minimal intervention, ensuring high accuracy in various applications. The microservices integrate seamlessly with platforms such as Cohesity, DataStax, NetApp, and Snowflake.
These microservices are particularly beneficial for developers working on AI agents, customer service chatbots, security vulnerability analysis, and extracting insights from complex supply chain data. By enabling high-performance, enterprise-grade inferencing, NeMo Retriever NIM microservices can supercharge AI applications with enhanced data accuracy and throughput.
Embedding and Reranking Models
NeMo Retriever NIM microservices consist of two main model types: embedding and reranking. Embedding models transform diverse data into numerical vectors, capturing their meaning and nuances, while reranking models score data based on its relevance to a given query. By combining these two models, developers can ensure the most accurate and relevant results for their AI applications.
The embedding models, such as NV-EmbedQA-E5-v5 and NV-EmbedQA-Mistral7B-v2, are optimized for text question-answering retrieval and multilingual embedding, respectively. The reranking models, including NV-RerankQA-Mistral4B-v3, provide high-accuracy text reranking capabilities. These models are now generally available and accessible through the NVIDIA API catalog.
Top Use Cases
NeMo Retriever NIM microservices offer a wide range of applications, from building intelligent chatbots and analyzing security vulnerabilities to extracting insights from supply chain information and enhancing retail shopping advisors. These microservices are also being integrated by various partners to boost the accuracy and throughput of their AI models.
For instance, DataStax has incorporated NeMo Retriever embedding NIM microservices into its Astra DB and Hyper-Converged platforms, while Cohesity is integrating these microservices with its AI product, Cohesity Gaia. NetApp is collaborating with NVIDIA to connect NeMo Retriever microservices to its intelligent data infrastructure, enabling seamless access to business insights without compromising data security.
Integration with Other NIM Microservices
NeMo Retriever NIM microservices can be used alongside other NVIDIA microservices, such as NVIDIA Riva NIM, which enhances speech AI applications. Upcoming models like FastPitch and HiFi-GAN for text-to-speech applications, and Megatron for multilingual neural machine translation, will soon be available as Riva NIM microservices.
These microservices can be deployed in various environments, including cloud instances from major providers like AWS, Google Cloud, Microsoft Azure, and Oracle Cloud Infrastructure. They can also run on NVIDIA-Certified Systems from server manufacturing partners like Cisco, Dell Technologies, Hewlett Packard Enterprise, Lenovo, and Supermicro.
Members of the NVIDIA Developer Program will soon have free access to NIM for research, development, and testing on their preferred infrastructure. Enterprises can deploy these microservices in production through the NVIDIA AI Enterprise software platform.
Image source: Shutterstock